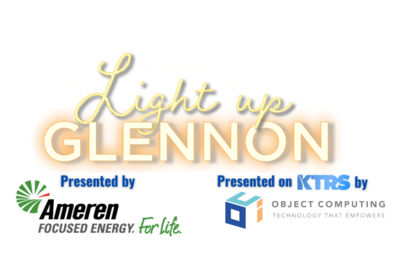
Illuminating the Holidays with Light Up Glennon
We believe in reinvesting in our communities through technology, thought leadership, and targeted financial investment. We are grateful for the opportunity to present Light Up Glennon on KTRS for SSM Health Cardinal Glennon Children’s Hospital and its beautiful holiday program.
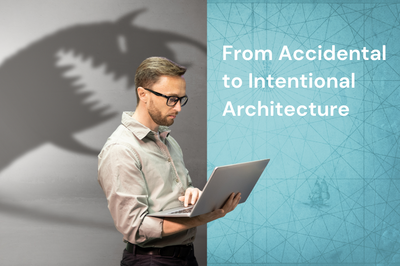
From Accidental to Intentional: Your Roadmap to Architectural Excellence
The first article of this two-part series discussed how to identify Accidental Architecture and the challenges it can cause an organization. We defined Intentional Architecture as a deliberate system architecture that is created from a set of goals, trade-offs, team structure, and constraints under which it’s built and maintained.
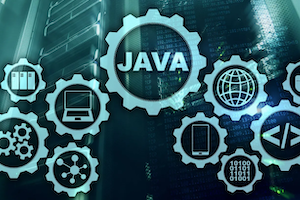
Maximizing Performance with Netty and Reactive Programming in Java
In the modern world of software development, building responsive and scalable applications is essential. This is especially true for cloud-based deployments, where keeping costs down while maintaining performance is crucial. Reactive programming, a paradigm centered on efficiently processing asynchronous events and data streams, can be an excellent choice for achieving this.
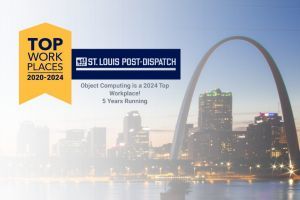
Object Computing Named a Top St. Louis Workplace Fifth Year Running
Object Computing has been named a Top Workplace for 2024 by the St. Louis Post-Dispatch. This is the fifth consecutive year Object Computing has received this honor. This list is based solely on employee feedback gathered through a third-party survey administered by employee engagement technology partner Energage, LLC.
An Open Letter to Our Open Source Communities
I am writing to share an update regarding our ongoing investment in Grails Framework, and to engage a dialogue around how all of us can work more collaboratively and intentionally to ensure that we keep the flames of our open-source innovations vibrant and everlasting.
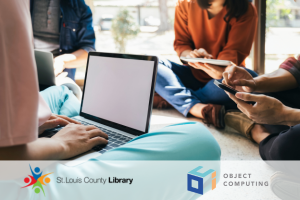
Object Computing Celebrates Debut of High-Tech Teen Creative Lab at St. Louis County Library
Object Computing, a technology consulting firm based in St. Louis, MO, joins the St. Louis County Library in celebrating the grand opening of the Clark Family Branch this summer, as announced in a recent press release by the Library. This milestone marks the exciting debut of the Object Computing-sponsored Creative Lab, a state-of-the-art hub designed to ignite creativity and foster tech exploration among youth.
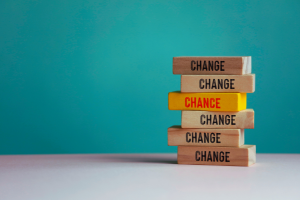
Navigating the Tech Landscape in 2024: Strategic Insights on Offshoring
By Garey Hoffman
As a technology leader immersed in IT consulting, I find myself reflecting on Gartner’s latest 2024 Forecasts of IT Spending. The projections indicate a noteworthy growth of 6.8% this year, a significant uptick from the 3.3% observed in 2023.
This part of the announcement gave me pause...