Case Study
AI App delivers maxium yields
Mobile app uses image analytics and machine learning to diagnose crop disease and provide accurate treatment recommendations in real time.
Growers find themselves attempting to battle crop disease with one-size-fits-all solutions that not only fail to address their specific needs, but often result in undesired side effects.
The Client
Evergreen is a company created to revolutionize the world of agriculture through trailblazing methods of harvesting science. The company, designed to be a natural and essential part of the agricultural scouting process, sets out to transform the traditional acre into an innovative Field of The Future.
The Challenge
-
Growers lack timely data to improve crop yields effectively.
-
Traditional scouting and drone monitoring methods are inefficient and prone to high error margins.
-
Manual data collection leads to delays and inconsistencies in identifying disease pressure.
-
Scalability of existing processes is critical to meet the demands of modern agriculture.
Our solution offers AI for routing of scouts, and Scientifically Defensible Artificial Intelligence that monitors for pathogen and disease pressure.
The Solution
-
Our team developed the Agtrinsic App, utilizing machine learning to identify subtle disease patterns.
-
The app analyzes nearly undetectable changes in plant height, ambient conditions, and residue through satellite imagery.
-
Weather data analytics inform drones and scouts of potential problem areas in real time.
-
Streamlined data collection enhances responsiveness and accuracy in addressing agricultural challenges.
The Outcomes
-
Increased efficiency in crop monitoring and disease detection.
-
Improved yield potential through timely interventions.
-
Enhanced decision-making capabilities for growers, leading to optimized agricultural practices.
Increase in Scouting Efficiency Compared to Traditional Methods
More Acres Scouted per Day than Traditional Methods
Faster Data Turn than Traditional Droning and Scouting Methods
Technologies and partners
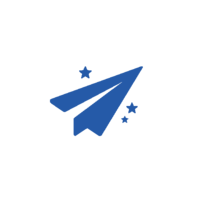
Consulting & Strategy
Outcome-focused, real-world practicality combined with courageous innovation
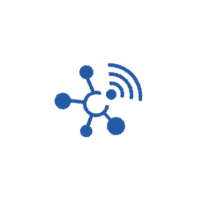
Technology & Transformation
Expert engineering from project initiation to deployment and beyond
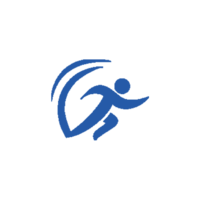
Technical Leadership & Support
Empowerment to scale your solutions long after our engagement has ended