It is generally accepted that successful businesses thrive by consistently making better decisions than their competitors, and the agriculture industry is no exception.
Through the application of artificial intelligence (AI) and machine learning (ML), growers can access increasingly sophisticated data and analytics tools, which enables better decisions, improved efficiencies, and reduced waste in food and biofuel production, all while minimizing negative environmental consequences.
Here is a list of innovative ways professionals in the agriculture industry are taking advantage of the powerful solutions now available through AI technologies.
The future of farming has never been so bright. There are an abundance of scalable technologies that reduce risk, improve sustainability, and place the grower in the center of predictively informed decisions … the new smart Ag is emerging.
AI-DRIVEN FIELD-SCOUTING INSIGHTS
Thousands of fields across the United States corn belt are harvested annually.
When it comes to routing equipment and teams to fields that are ready for them, there is a limited window of opportunity. Having the right equipment and manpower in the right place at the right time for optimal scouting presents a significant challenge for large agricultural operations with vast acreages to monitor and manage.
Our experienced engineers have built a system of machine-learning algorithms that consolidate and process data from multiple sources, including geospatial and weather data, to perform high-resolution imagery and vector analyses for hundreds of fields throughout the Midwest.
The results can then be shared with customers through a modern digital portal that connects up-to-the-minute field data to analytical models that deliver dynamically calculated insights.
Yield Prediction and Quality Assessment
Through the application of ML technology, a farmer can log into a customized dashboard on a computer or tablet and access an accurate assessment of the harvestable versus non-harvestable acres on a given day. The weight and maturity of harvestable crops can also be measured and predicted.
Additionally, using a variety of technologies, including image analysis, crops can be evaluated both before and after harvest for the presence of desirable features, extent of damage (if applicable), nutritional makeup, and other factors that may impact the ultimate viable yield and product price.
Crop Disease and Weed Detection
ML-driven image processing allows farmers to rely upon digital tools to recognize weed species and to determine which crops are healthy and which ones are infested with disease caused by fungi, bacteria, or viruses.
The ability to identify weeds with digital tools makes it possible to train mechanical devices (robots) to pull weeds from fields, protecting the environment from damage caused by pesticide use and saving farmers time, effort, and money.
Additionally, digital applications that can evaluate crops for disease can also provide an accurate disease diagnosis and recommend an optimal treatment plan.
This technology helps farmers avoid settling on a one-size-fits-all solution that not only fails to address a specific disease, but may inadvertently cause undesirable side effects, such as pollution or bee-population reduction. It also allows the companies that manufacture crop disease treatment products to better serve their customers.
Species Identification
Many plants have similar leaf compositions, colors, and shapes, making it difficult to label them using the human eye. Farmers can now rely upon ML to assess complex patterns and accurately identify related plant and weed species.2
Digital identification of plant species saves farmers time, allowing them to increase productivity in other critical areas.
“AI isn't the solution, it is the secret ingredient to a smarter outcome.”
— Product Manager, ML-Insights, Andrew Montgomery
Real-World Use Case
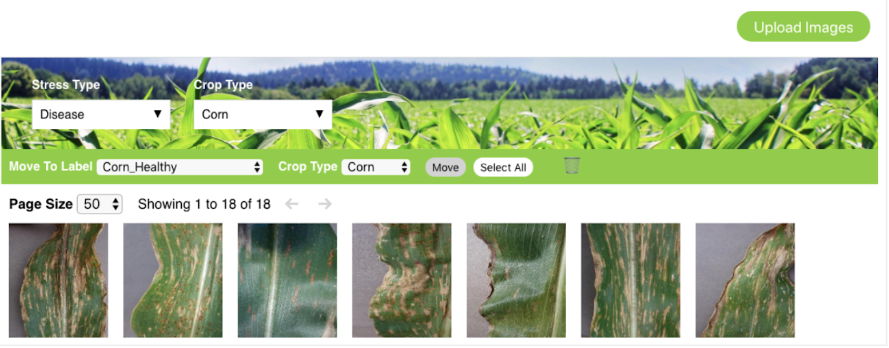
Object Computing built a mobile solution that allows farmers to photograph diseased plants and receive accurate diagnostics and product recommendations instantaneously.
Farming Summary
Today's farmers rely upon ML-enhanced technology to:
- Map and estimate yields
- Better meet demand without unnecessary waste
- Make smarter harvesting and pricing decisions
- Identify and automatically remove harmful weeds
- Find and treat crop disease with targeted solutions
- Accurately classify weed species
- Increase productivity, save time, and operate more economically
- … and the list continues to grow!
Ranching
Those who work with livestock are also experiencing time and cost savings as a result of ML-driven technology.
ML technology currently improves farming and ranching operations in a variety of areas, including livestock health maintenance, dairy and egg production, animal herding, and selective breeding.
Livestock Health Maintenance
ML helps farmers maintain happy and healthy herds of cattle.
In one use case, a company in Amsterdam uses sensors to monitor cow behavior. ML analysis of the gathered data predicts fertility patterns, diagnoses eating disorders, and alerts farmers to signs of heat stress.4
In another example, a dairy cow's health status can be evaluated by applying deep learning algorithms to images of white blood cells extracted from the cow's blood or milk. This process provides indications of certain health issues earlier than simple observation provides. Farmers can then initiate appropriate wellness measures before antibiotics become necessary.4
Dairy and Egg Production
ML-driven data analysis also helps ranchers optimize operations to more accurately and efficiently manage production of milk, eggs, and other foods.2
For example, the same technology that gathers and analyzes data on dairy cow activity also allows farmers to make operational decisions that improve milk output by up to 30%.5
Animal Herding
Autonomous robots aren't just monitoring fields of crops for weeds and diseased plants. They're also being trained to herd cattle and sheep.
In addition to physically herding animals toward a desired destination, these ML-driven devices can haul heavy objects from one place to another and cooperate with drones to relay critical information to farmers.6
Selective Breeding
Selective breeding involves the use of genetic data to optimize livestock pregnancy rotations and encourage the perpetuation of favorable traits, such as milk quality, disease resistance, fertility, and more.
Selective breeding is not new. Ranchers have relied upon observable factors to produce livestock lines that possess desirable characteristics for centuries. Today, with the assistance of ML-supported sensors and applications, a vast amount of data regarding genetic molecular markers, environment, feed makeup, birth patterns, and more can be analyzed, and ranchers are able to make livestock-mating decisions with significantly more accurate results.
Ranching Summary
Ranchers rely upon ML-enhanced technology to:
- Analyze livestock behavior patterns and provide better care and feeding recommendations
- Diagnose disease and preemptively apply appropriate treatments
- Optimize milk and egg production
- Herd cattle and sheep
- Perform manual labor
- Evaluate and communicate farm-condition data
- Predict fertility patterns and improve breeding selection to produce hardier stock
- Increase productivity, save time, and operate more economically
Agronomy, Breeding, and Biotechnology
Agronomy, breeding, and biotechnology include practices that help improve agriculture operations and outputs, including water and soil management, hybrid plant optimization, and sustainable agrochemical production and application.
Water and Soil Management
Through ML-assisted analysis of precipitation and evapotranspiration (the process by which water transitions from soil and plant transpiration to the atmosphere), technologists develop more efficient resource management procedures and irrigation systems.2
ML is equally well equipped to analyze data regarding soil conditions, including moisture level, temperature, and chemical makeup, all of which have an impact upon crop growth and livestock well-being.
Plant Breeding
As with humans, plants' characteristics are determined by their genes. Certain genes help plants absorb water and nutrients better than others, while others help them fight disease more effectively. Some genes even affect how a plant may end up tasting!
An entire industry revolves around developing commercial seed products that combine the best features of various plant strains.
Without the aid of ML-based technology, a single hybrid development cycle can take scientists seven or eight years (although this is still faster than the speed at which nature performs the process!).
By evaluating masses of data on plant performance in various conditions over time, ML algorithms help scientists better optimize the identification of biotech traits needed to profitably increase yields, given the likelihood of harmful environmental factors, such as unfavorable weather conditions and insect populations, in a given season. This optimized use can also improve the longevity of these hugely beneficial and expensive-to-create biotech traits by reducing resistance buildup.
Simply put, ML helps scientists make predictions regarding which gene combinations will lead to desirable traits in new plants, providing an excellent starting point for developing hardier (and possibly more flavorful!) plant species.
“By incorporating active learning [a type of machine learning], we can create models that offer the potential to reduce and improve the expensive research experiment footprint required for product characterization and commercialization, and also provide valuable insights on predicted product deployment targets.”
— Object Computing Director of Machine Learning Solutions, Dr. Xiao Yang
Agrochemical Production and Application
One of the key strengths of ML technology is predictive analysis. Companies that develop chemical and biochemical products for farmers and ranchers, such as pesticides, crop disease treatments, antibiotics, microbials, and more, rely upon ML to assist them in ensuring that product efficacy is maximized and that the environmental impact of the products they place on the market is minimized.
Additionally, as mentioned earlier, ML simplifies and streamlines the entire crop-disease identification, diagnosis, and treatment process.
The mobile app capable of providing farmers plant disease diagnoses in real time also delivers product recommendations. Order fulfillments can then be handled via ML-assisted digital processes, and spray drones programmed to recognize those crops that require treatment can pinpoint delivery of the right products to the right plants without disturbing neighboring, healthy or unaffected plants.
Plant disease isn't the only threat to crops and livestock that ML helps professionals combat. By using a similar image-analysis algorithm, pest control companies provide their associates a reliable, real-time tool to identify bugs, allowing them to provide targeted extermination services.7
Agronomy, Breeding, and Biotechnology Summary
Technologists rely upon ML-enhanced technology to:
- Analyze and optimize water and soil resources
- Develop better hybrid plant species
- Reduce harmful environmental impacts of pesticides and other products
- Remotely diagnose crop disease and provide targeted solutions
- Deliver crop disease treatments and pesticides with pinpoint accuracy via spray drones
- Identify pests and provide targeted solutions
- Increase productivity, save time, and operate more economically
Takeaway
The opportunities to enhance agriculture operations using ML and AI are by no means exhausted; in fact, we currently stand at the beginning of one of the most impactful chapters the agriculture industry has ever seen.
Information – and more importantly insight – is essential to modern agriculture, from the creation of new hybrid and varietal products, to the placement of products in the correct management zones, to capturing value at harvest time.
As technology advances, industry experts and technologists are working together to imagine solutions that were nothing but science fiction even a few years ago.
Is it time to reimagine what's possible for your business?
1 Transforming Field Data Into Meaningful Insights | 2 Machine Learning in Agriculture: A Review | 3 Podcast: The Age of Digital Agriculture - Seed Advisor | 4 Herd of AI Startups Milking the Internet of Cows | 5 Using TensorFlow to keep farmers happy and cows healthy | 6 Even sheepdogs aren’t safe: A new robot can herd animals on its own | 7 AI, Machine Learning Blossom in Agriculture and Pest Control
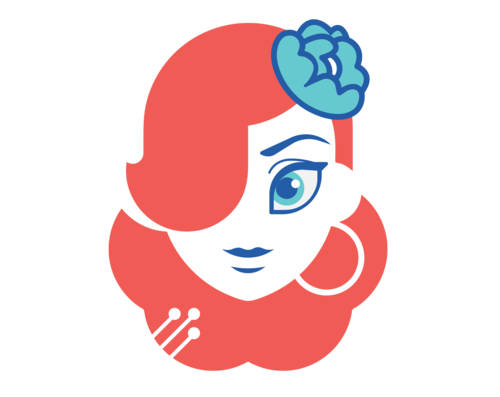
Meet the Alyce.ai® Accelerator
The Alyce.ai accelerator significantly accelerates the shift to predictive and optimized interconnected decisions for farmers, retailers, and agri-businesses, driving increased profitability and sustainability.