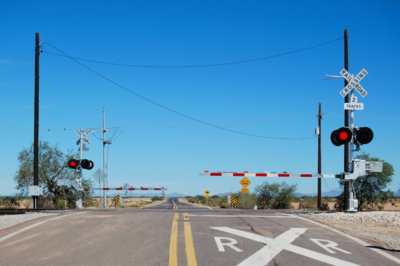
By Samuel Vanfossan and Allan Trapp
As an integral part of modern critical infrastructure and pivotal to the transportation of goods in many developed countries, rail carriers assume responsibility for thousands of miles of track that must be monitored and maintained. Performing these duties adequately is paramount to regulatory compliance and safety. However, the processes to conduct these operations are generally slow, manual, and resource-intensive. These inefficiencies can lead to backlogs and compromise safety.
The solution lies in building the right tech stack. By harnessing the power of cloud computing and machine learning (ML), we can extract rich insights from LiDAR (Light Detection and Ranging) data. Let’s dig deeper into the problems and how our solutions can provide railroads with vital and timely information for maintenance and intervention.
Challenges in Vegetation Management
One critical area requiring improvement is vegetation management at railway crossings. For safety reasons, the railway responsible for a crossing must ensure the area around it is free of vegetation that could obstruct visibility. When this vegetation management is done poorly, vehicle passenger and train operator sightlines can become obscured, leading to collisions and other accidents.
The manual and time-consuming process to complete this critical responsibility requires dispatching crews or contractors to take measurements and make assessments. Plus, the costs associated with conducting and validating manual assessments can be prohibitive, making it impossible to do with enough frequency to adequately manage vegetation encroachment. A means to expedite this process could serve to greatly enhance rail crossing safety, ensuring that visibility-obscured crossings are identified and remedied in a more timely manner.
LiDAR Technology and Its Potential
Fortunately, advancements in technology offer promising solutions. LiDAR is a popular tool for gathering data on trackside objects, including vegetation. However, the sheer volume of LiDAR data can be overwhelming, often remaining unused because there’s no easy way to interpret and analyze it.
Unlocking the Value of LiDAR Data
While LiDAR data holds undeniable value for railroads, it’s a tough nut to crack. It contains a massive amount of detail about trackside objects, making analysis challenging. However, if we can properly analyze this data, it can significantly speed up many tasks for rail carriers. This translates to both improved track safety and reduced resource needs for maintenance.
A Practical Example: Streamlining Vegetation Management
A specific application of this tech stack is a tool we designed to manage railroad-crossing vegetation. This tool leverages:
- LiDAR data collected from vehicles already using the railway.
- Cloud computing for secure data transfer and storage facilities, along with the processing power and scalability required to perform complex calculations and assessments systematically. The computational power and integrability of cloud services also enable the rendering and display of meaningful visualizations and reports based on the data analysis.
- Machine learning algorithms for automated insight generation, eliminating the need for manual inspection. This enables rapid assessment of large datasets, pinpointing critical issues and recommending targeted actions.
- Asterisms, a vendor-neutral platform for interactive data visualization.
The tool analyzes LiDAR data in conjunction with open-source geospatial data to:
- Detect and classify vegetation within designated compliance zones.
- Identify potential compliance violations related to vegetation growth.
- Quantify the extent and type of vegetation occluding the view.
LiDAR in Production: Road-Rail Intersection Sightline Compliance
By combining the gathered LiDAR and open-source geospatial data with powerful ML techniques, the tool detects and classifies vegetation within specified compliance zones around railway crossings. The analysis culminates in identifying compliance violations and details the magnitude and variety of the vegetation occlusion. These insights, both qualitative and quantitative, provide a meaningful evaluation of the crossing’s sightline compliance and can be used directly to identify crossings needing vegetation management.
This tool goes beyond simply identifying vegetation by providing:
- Detailed breakdowns: The tool calculates an “occlusion proportion” for each zone, revealing the overall blockage percentage and breakdown by vegetation height.
- Interactive 3D visualizations: Users can virtually explore 3D maps of crossings, complete with surrounding vegetation and objects.
- Prioritization for resource allocation: Capable of analyzing thousands of crossings in a matter of minutes, the tool allows users to quickly identify and prioritize the most critical compliance violations and determine the necessary remediation resources.
- Remote inspection: Through the supplied 3D visualizations, users can remotely search and inspect crossings of interest to verify reported statuses.
All of this occurs in the browser, providing an automated and centralized toolkit instead of a formerly manual and fragmented practice.
The Utility of Using Your LiDAR Data
The successful utilization of LiDAR data means reduced manual work for rail carriers while pursuing maintenance standards and compliance. Not only is the manual workload (and associated resource cost) of data collection mitigated, but the effort required to complete assessments about this data is reduced. Nesting LiDAR data within the detailed tech stack allows targeted insights to be generated automatically and much more expeditiously.
Accordingly, the time to insights is greatly improved; the delta between data collection and the realization of actions to be taken becomes much smaller. This throughput enhancement then allows operators to dispatch remediation resources in a more timely manner. The generalized reduction in procedural effort and time lets assessments be conducted more frequently, keeping a more-present eye on the status of railway conditions.
The real power of this technology lies in its speed. As multiple assessments can be completed quickly, action item prioritization is also enabled. Considering a large number of identified tasks to be completed, the most pressing or impactful can be selected to receive correction efforts first. This not only helps railways comply with regulations, but more importantly, it keeps everyone safe.
Looking Ahead
This technology represents a significant step forward. We are already developing ways to use the same basic architecture with LiDAR data for many other applications, both on and off the rails. Early tests show it can be used to assess ballast quality, monitor the health of the tracks and ties, identify objects encroaching on the tracks, and even evaluate tunnel quality.
The integration of LiDAR, cloud computing, and machine learning holds immense potential for a wide range of applications, not just in railways but across various industries.
See a demo of this tool and learn more about our LiDAR expertise.
Samuel Vanfossan, PhD, is a Data Scientist at Object Computing, responsible for the design and implementation of machine learning and operations research solutions. His interests include the application of artificial intelligence to geospatial data and the intersection of machine learning and optimization.
Allan Trapp, PhD, is the Managing Director of Data Science at Object Computing. He leads a team of data scientists and engineers who craft ML business solutions across diverse industries including agriculture, transportation, finance, and life sciences. Data-driven actions are his passion and motivate his research on agricultural management zones and the carbon-offset marketplace.